Discovering And Interpreting Conceptual Biases (DAICB)
The DAICB is a tool to interactively compare the discovered biases between two attribute concepts inherent in large textual datasets taken from the internet, as captured by Word Embeddings models. It is an extension over previous work presented at ICWSM, which can be accessed here.
Language carries implicit human biases, functioning both as a reflection and a perpetuation of stereotypes that people carry with them. Recently, ML-based NLP methods such as word embeddings have been shown to learn such language biases strikingly accurately. This capability of word embeddings has been successfully exploited as a tool to quantify and study human biases. However, previous studies only consider a predefined set of conceptual biases to attest (e.g., whether gender is more or less associated with particular jobs), or just discover biased words without helping to understand their meaning at the conceptual level. As such, these approaches are either unable to find conceptual biases that have not been defined in advance, or the biases they find are difficult to interpret and study. This makes existing approaches unsuitable to discover and interpret biases in online communities, as online communities may have different biases from mainstream culture which need to be discovered and properly interpreted. Here we propose a general, data-driven approach to automatically discover and help interpret conceptual biases encoded in word embeddings. We apply this approach to study the conceptual biases present in the language used in online communities and experimentally show the validity and stability of our method.
Explore the data for
Click on any of the cards and explore different interactive approaches to discover stereotypes and biases found in the selected dataset.
Semantic Categorisation of Conceptual Biases
The figures below show an overview of the conceptual biases found in the dataset, obtained after tagging every cluster in the partition of conceptual biases with the most frequent semantic fields (domains) among its words. This allows us to get a general idea of the nature of the conceptual biases discovered for (left pie) and (right pie) in this community.
Detailed Dataset Conceptual Biases
This section shows the details of the conceptual biases for (top table) and (bottom table) discovered in the dataset. By ordering the clusters by the different properties, we are effectively ranking the clusters based on the different startegies presented in the paper Rf, Re and Rs.
Rankings reported in the paper for and
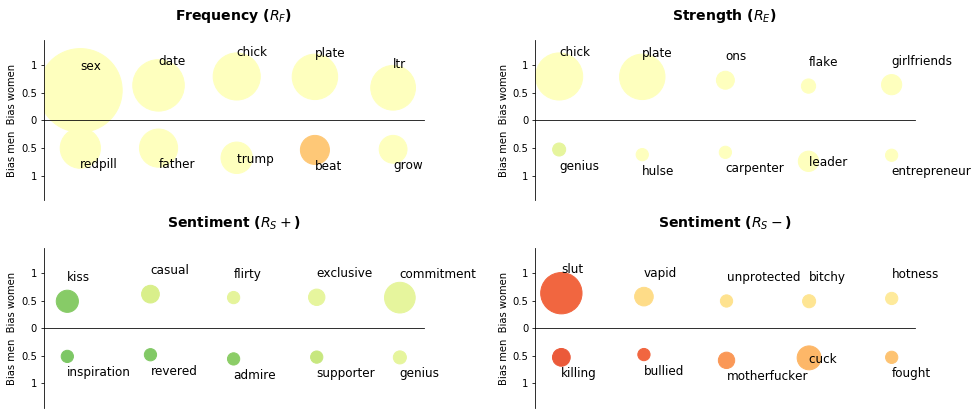
# | Words | Total Frequency | Avg. Salience | Avg. Bias | Avg. Sentiment |
---|
# | Words | Total Frequency | Avg. Salience | Avg. Bias | Avg. Sentiment |
---|
Most Frequent Salient Words
The word clouds presented below show the most salient words biased towards and (left and right, respectively) in the selected dataset, that is, these words more often found in and related contexts. The size and color of each word corresponds with its frequency, bigger means more frequent. For details about each word, see section Detailed Dataset Word Biases.
Detailed Dataset Word Biases
This section shows the details of the most salient and biased words for and (left and right, respectively) in the dataset.
# | Word | Sal | Bias | Freq | Sent | POS |
---|
# | Word | Sal | Bias | Freq | Sent | POS |
---|
Word Distributions of Biases
Explore the bias and frequency distributions of all biased words in the dataset in two bar plots; -biased words are shown on the top bar plot and -biased words on the bottom. By comparing the distributions, one could observe the differences between genders/religions in the dataset (depending on the commnuity explored). For instance, in The Red Pill, although men-biased words are more frequent, women-biased words hold stronger biases.
Bias Polarity
Explore the sentiment of the most salient and biased words for and (left and right, respectively), classified in 7 categories ranging from positive to negative.
Word Embedding Space
Explore the distribution of salient and biased words in the embedding space as learned by a machine learning algorithm, represented in the two principal t-SNE dimensions.
Concept Embedding Space
The figure below shows the distribution of and concepts on the embedding space for the selected dataset, presented in the two most informative t-SNE dimensions.